The Tech
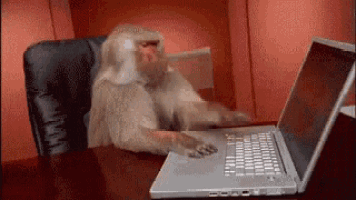
The Fun Stuff
These are technologies that I have worked with, and have built an opinion about.
I do not consider myself an expert in these technologies.
However, I do feel that I have a holistic view of the entire ecosystem.
I prefer functional code to OOP, ergo Go and scripting languages. More generally, for most developer problem-domains the bottleneck is IO. E.g. API calls, DB calls, disk writes, etc. As such, in most cases Node is excellent. It’s quite fast, and the ergonomics are top notch. However, it does force developers into a multi-process paradigm (e.g. Kubernetes). For problems that require multithreading over multi-process (e.g. Tensorflow, CSS rendering, fintech pricing), Node is not a good choice, and a second language is reuqired. Similar to Google’s Python + C++ architecture. More on Rust below!
I’m passionate about CI and CD. Good CICD directly translates to developer productivity and team confidence.
On the CI side, imagine being able to test everything on every commit (more on testing below). I wrote many of Kindred’s pipelines, including in-pipeline dockerised integration tests (using docker-in-docker), load tests, multi-pipeline E2E tests with ephemeral environments (using kubernetes-hnc), and lots of other goodies. Good CI changed the way I hire QAs.
On the CD side, sports betting is a highly regulated industry. There is a joke at Kindred: “Kindred is a compliance company that occasionally takes a bet”. This significantly complicates our deployment workflow. Gitflow, gitops, government audits, gated workflows. We are still wrangling with this and iterating towards an “ideal” workflow.
In the relational space, I spent a fair bit of time with MySQL and PostgreSQL. Things like indexes (composite, hash, functional), keys (primary, foreign, unique), migrations, `explain analyze`
, isolation levels, efficient bulk queries, and avoiding ORMs (cognitive overhead, lazy/eager loading performance).
In the NoSQL space, I built the startup’s MongoDB cluster (shard keys and all). These days, I prefer to use a hybrid approach: postgres + jsonb (credit to Emil Koutanov). I also built the startup’s Solr instance and queries. Solr’s “edismax” queries were incredible. Fantastic tech.
The startup began with a php web app and an iOS app. The most senior engineer (Ruslan Starikov) and I had to make a decision on what to do next. This was at the time of Angular 2.0. We didn’t like Angular’s monolithic approach, the breaking changes of 1 => 2, nor the combination of html + js files. Conversely, we liked that React is in essence just a library for writing html in javascript. This is representative of my general approach to engineering, i.e. the Unix Philosophy. It also allowed us to roll the entire app into one “binary”, making hosting super easy. From there we built up the ecosystem with state management (this was the early days of Redux, so we wrote our own Flux implementation). Then routing (with react router) – we hit some walls with SPAs and Google SEO. And so on.
We also considered react-native at the time we made the decision. I was against react-native for two reasons: technicals and politics. On a technical level, these translation layers tend to be janky (think Xamarin). Apple may change its APIs as it sees fit, and Facebook may or may not keep up. On a political level, Facebook and Apple have a relationship of convenience and that may change tomorrow. It’s hard to tell where that would leave react-native. Keep in mind that Apple has a history of nuking software from their walled garden (think Adobe Flash). It’s when we wrote a proof of concept using Electron.js that we became confident that we are on the right track.
In my experience, cloud has had two major benefits.
One: the ability for teams to own their platform. This has led to many political issues in the past (infra teams, devops teams, network teams, ownership).
Two: infra as code. Developers got build more robust, reproducible platforms as a result. If a similar level of autonomy could be achieved on prem, with something like OpenStack, I wouldn’t be against it. But I have not seen it done well, so far.
I feel relatively new to the world of observability, and SRE. There were some failures at Kindred before we got it right. We tried Elastic APM but the infra team hated maintaining it. We tried Instana, but it was $1m/year and not particularly nice. Finally, Kindred started doing observability in anger in 2024. OpenTelemetry libraries are used for language-agnostic, platform-agnostic data collection (no rework when changing back-ends). The back-end is composed of the Grafana stack (Tempo, Loki, OnCall). Metrics are owned by applications, and pulled by Prometheus. Metrics provide coarse-grain statistics, and traces provide single-journey level granular info (timing, distributed traces). Currently we are doing 100% sampling, as we figure out something smarter. We use Elastic for logging, as we move to Loki. Loki is free, and simple under the hood, essentially acting as “distributed grep” (Aidan Hall). Overall, the observability journey has been very rewarding, but there is a lot left to learn. Probably more than any other topic on this page.
The majority of my experience with message-oriented-middleware has been with Kafka. Things like:
– partial sort order & msg keys
– headers & versioning
– compression, LZ4
– batch operations, de-duplication, tweaking batch size
– (we didn’t get to parallel processing of a batch yet)
– kafka-ui, kowl
– outbox pattern (we didn’t use it)
Emil Koutanov wrote a book on Kafka, so a lot of questions go in his direction.
This ties into the CICD point above. Applications are packaged as docker container and helm charts published to a registry. At that point, the application can be deployed using a variety of CD tools. As of 2024, we are looking at Argo CD. Importantly, this entire stack can be run locally, giving developers a fast feedback loop, and keeping their development platform close to production. Istio has also been helpful in this regard, by making transparent service URLs. E.g. `http://myservice.mynamespace/api` will work in all environments, including local.
Testing software has become a major undertaking at Kindred, as we scale out the platform to millions of users. Performance testing in particular. Tools like k6 are used for simple API load tests. However, the platform is intentionally asynchronous where possible, allowing it to scale, and to have robust fault tolerance. As such, some domains may only have one API for public consumption, whereas all other services in the domain communicate asynchronously. Load testing these domains requires asynchronous load tests, which has been a fun challenge. E.g. load up a topic, start a stopwatch, watch the consumer lag go down to zero.
This also raised the question of reproducibility, which ties into the CICD and Orchestration points above. Due to the use of k8s, we are able to deploy a whole domain to an ephemeral environment in roughly five minutes, and then tear it down. This allow us to have reproducible “integration level” load tests of the platform, giving developers a high level of confidence about their changes.
Finally, we are now working on storing the results of these load tests in Prometheus/Grafana (see the Observability point above). Tools like k6 are compatible with Prometheus off-the-shelf. This means that we can chase performance regression retroactively. I.e. if we see that performance fell of a cliff on the first of January, we can check what was committed.
Testing software has become a major undertaking at Kindred, as we scale out the platform to millions of users. This includes:
- classic unit tests with a high coverage rate
- in the past, I had a gate configured to fail a build if coverage drops, I need to bring that back
- static code analysis with sonarqube, gated on critical severity issues in the build pipeline
- security scanning using sonarqube and snyk, gated on critical severity issues in the build pipeline
- small integration tests and load tests in the build pipeline using docker containers and docker-in-docker
- system-level integration tests triggered by the build pipeline, using ephemeral infrastructure (DBs, kafka topics, etc) and ephemeral k8s namespaces
- basic smoke tests in the form of k8s liveness/readiness probes
During my time at lumio, we initially wrote a product search engine on top of Solr, a technology similar to Elasticsearch, and also built on top of the java Lucene library. This led us down the rabbit hole of edismax queries, boosted fields, language parsing (tokenization, stemming, etc), and we built an appreciation of how hard working with multilingual freetext can become. Our search engine was awesome though, easily better than Asos and the like.
As we pivoted, we collected terrabytes of text data. After some intensive googling, we came to the conclusion that a semi-structured approach was the only way to process such data with any degree of consistency and success. This led us down the Natural Language Processing (NLP) path. NLP uses a supervised AI model in order to extract data. We leveraged an open-source NLP library called SpaCy to do this. It proved incredibly effective at extracting structured data.
We were super excited to keep pushing with image recognition (specifically, object recognition and facial recognition) in order to extract further data, but our journey was cut short as we ran out of cash. It felt like we only dipped our feet in the AI domain.
– databse migrations
– twelve-factor apps
– ephemeral environments
– dependency injection, service providers
– facades (slf4j, open telemetry, open features)
– statically-defined contracts (open api)
– async/await workflows (IO is the biggest bottleneck)
– code quality (formatting, linting, static code analysis, sonarqube)
– testing (see above)
– fully self-contained component tests (act as documentation)
– security (trivy, snyk)
– trunk based development
– layered architecture
– load tests on day zero
I am a Mozilla guy from the 90s Netscape days. Firefox is written in C++, and Mozilla decided that C++ was not fit for purpose. Namely, the highly parallelised CSS rendering engine. As a result, Rust was born. To me this is significant. Rust was not built as an academic exercise, nor a convenience, but as a solution to a fundamental limitation of C++. This is why it has potential. If you want to know more about the motivation, look into Firefox Quantum. I drove some early adoption of Rust at Kindred. However as a manager, it has been delegating, rather than hands-on.